Learn what AI driven environmental data analysis can do.
The KISTE project aims to exploit recent developments in artificial intelligence – especially deep learning methods – for sound environmental data analysis.
Find your CourseFeatured Courses
Computational aspects of AI for environmental sciences
Understanding how to deal with Earth system data is a must for environmental data scientists. Here, you learn the basics and important modern concepts to efficiently cope with very large data volumes.
Start CourseAll Courses
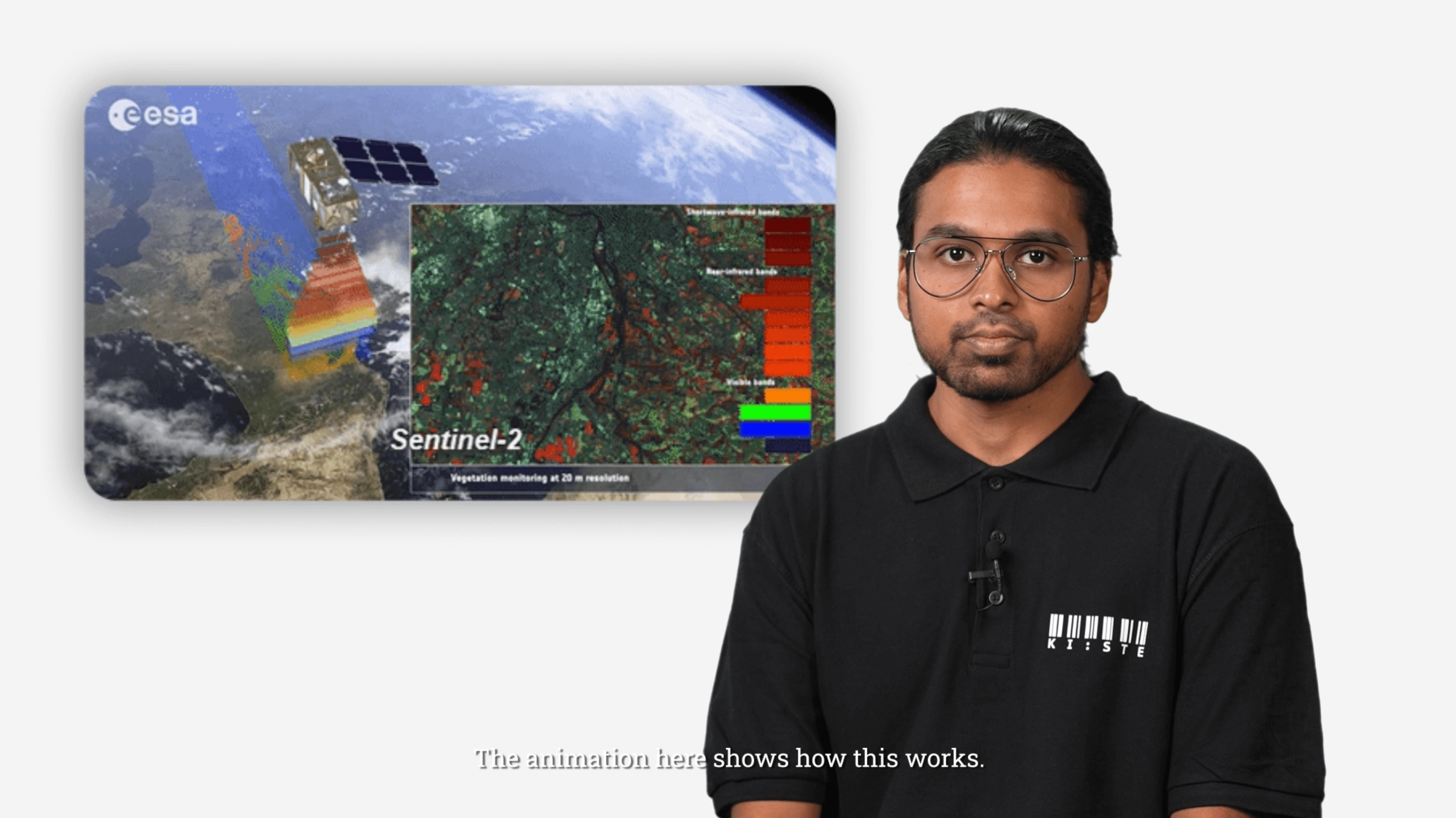
AI for landcover classification
Dive into remote sensing images, benchmark datasets, contrastive self-supervised learning, and explainable machine learning for Landcover Classification. Discover geospatial datasets in remote sensing and learn handling datasets for effective remote sensing application.

AI methods
Explore AQ-Bench, understand neural networks and random forests, and acquire tools to assess prediction quality. Synthesize insights on models and datasets for a holistic understanding. Participate in a concise exploration of ML model representation and dataset analysis.
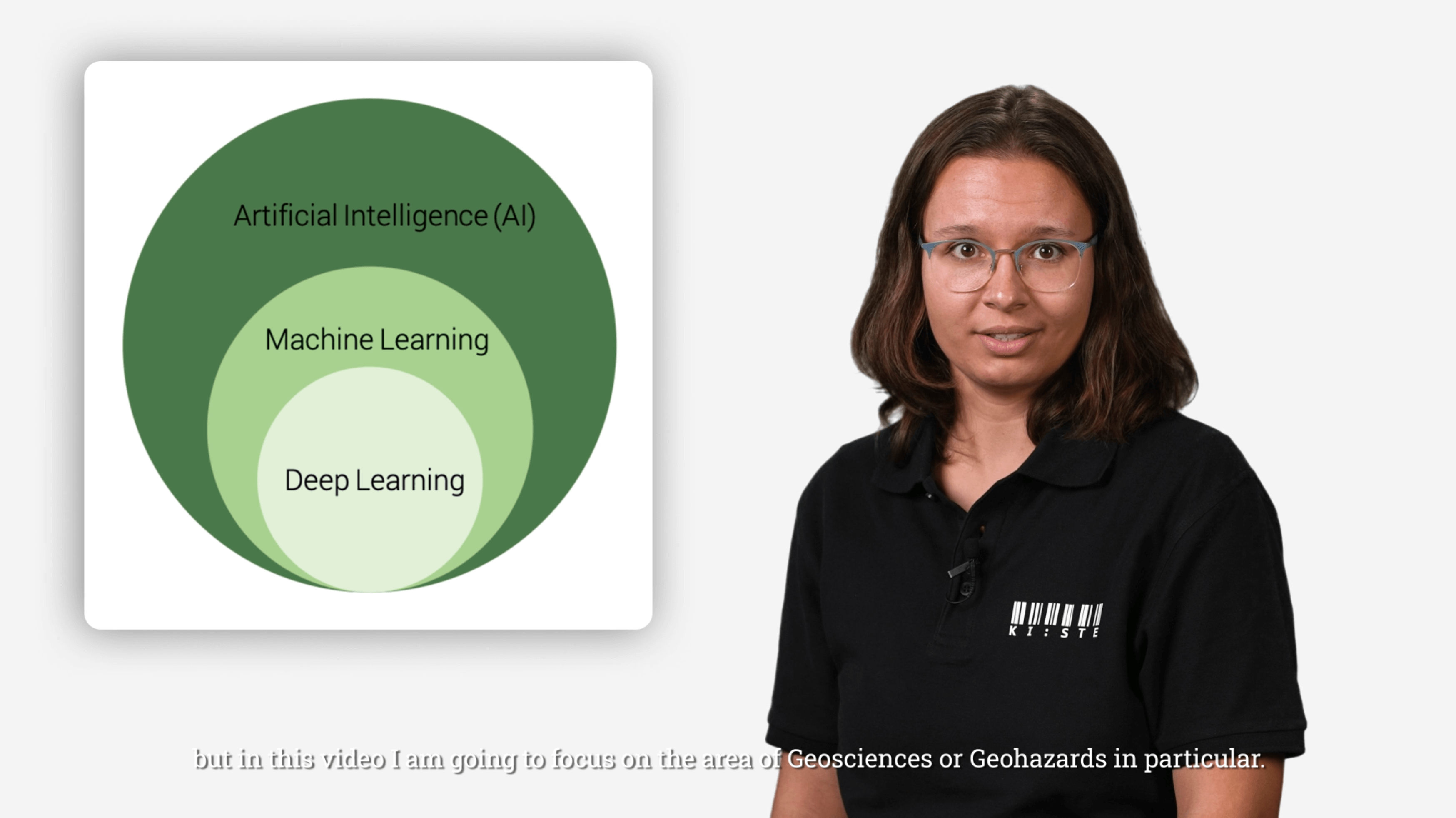
AI for geohazards
This course offers an introductory exploration of AI methods in Geohazards research with a special focus on machine learning-based landslide susceptibility mapping
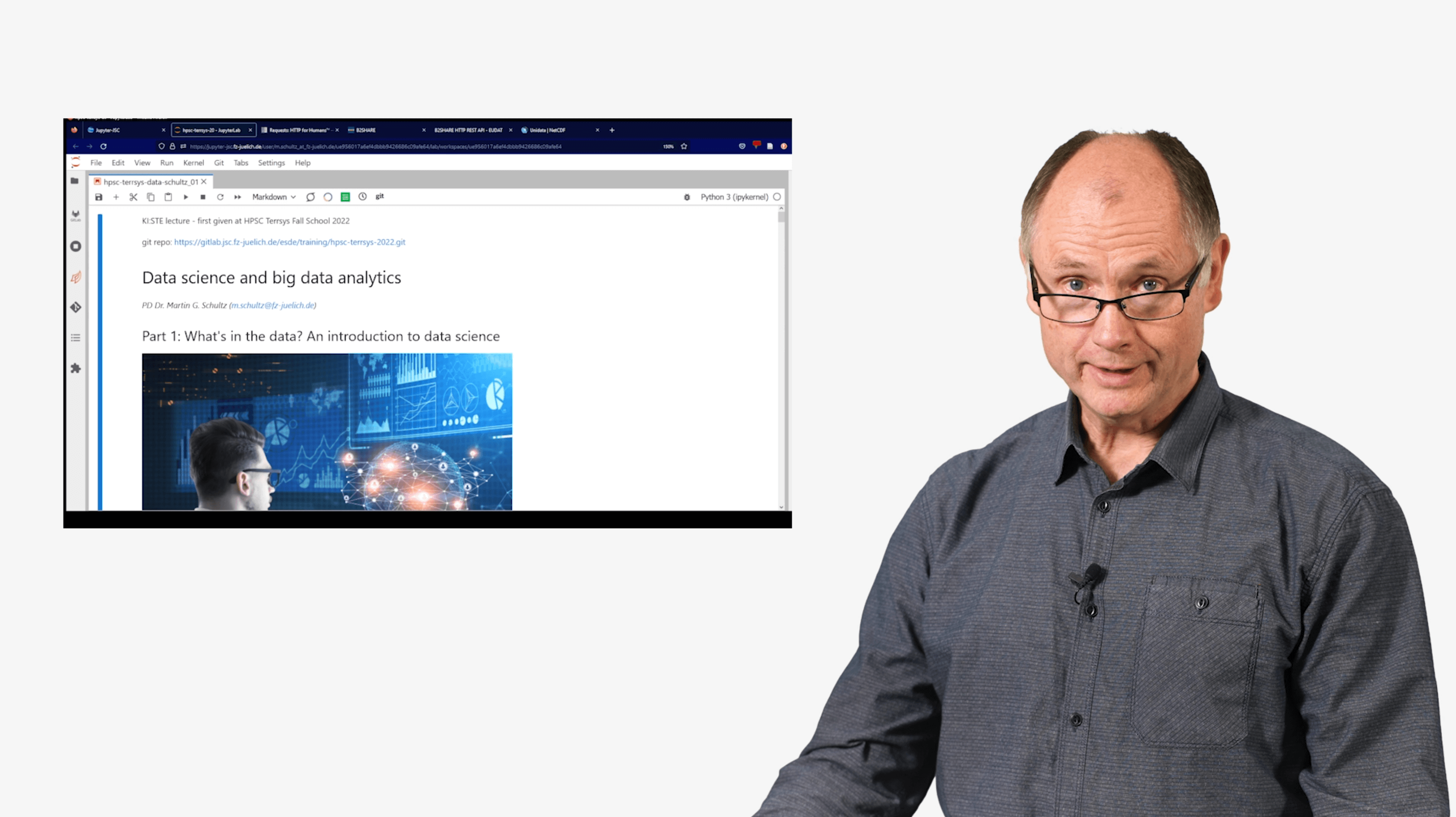
Computational aspects of AI for environmental sciences
Understanding how to deal with Earth system data is a must for environmental data scientists. Here, you learn the basics and important modern concepts to efficiently cope with very large data volumes.
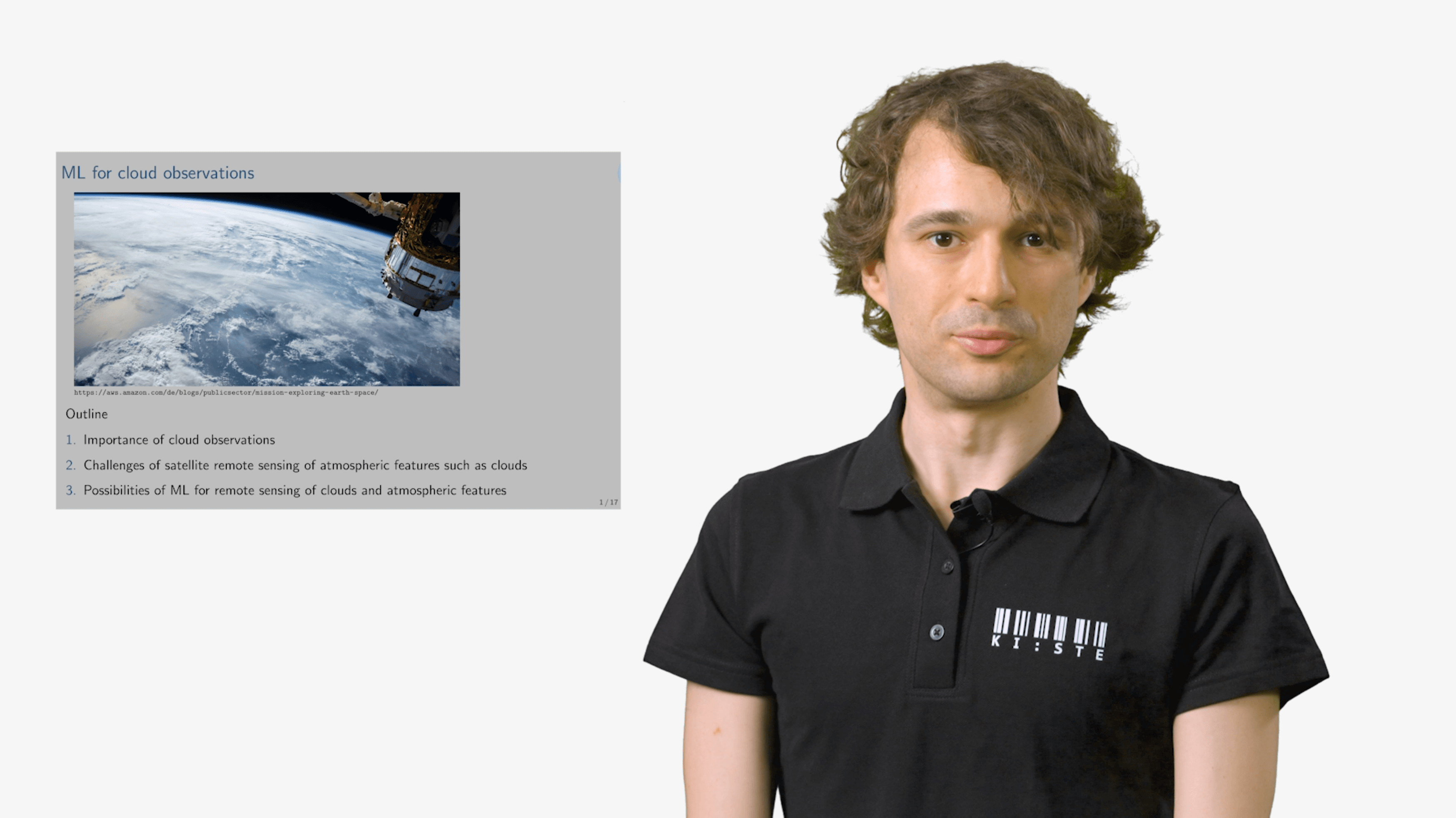
AI for cloud classification
Explore the world of cloud dynamics through AI. Uncover structural insights, climate implications, and overcome dataset challenges. Discover neural architecture and optimization. Refine your insights of AI-driven cloud classification for weather prediction and climate modeling.
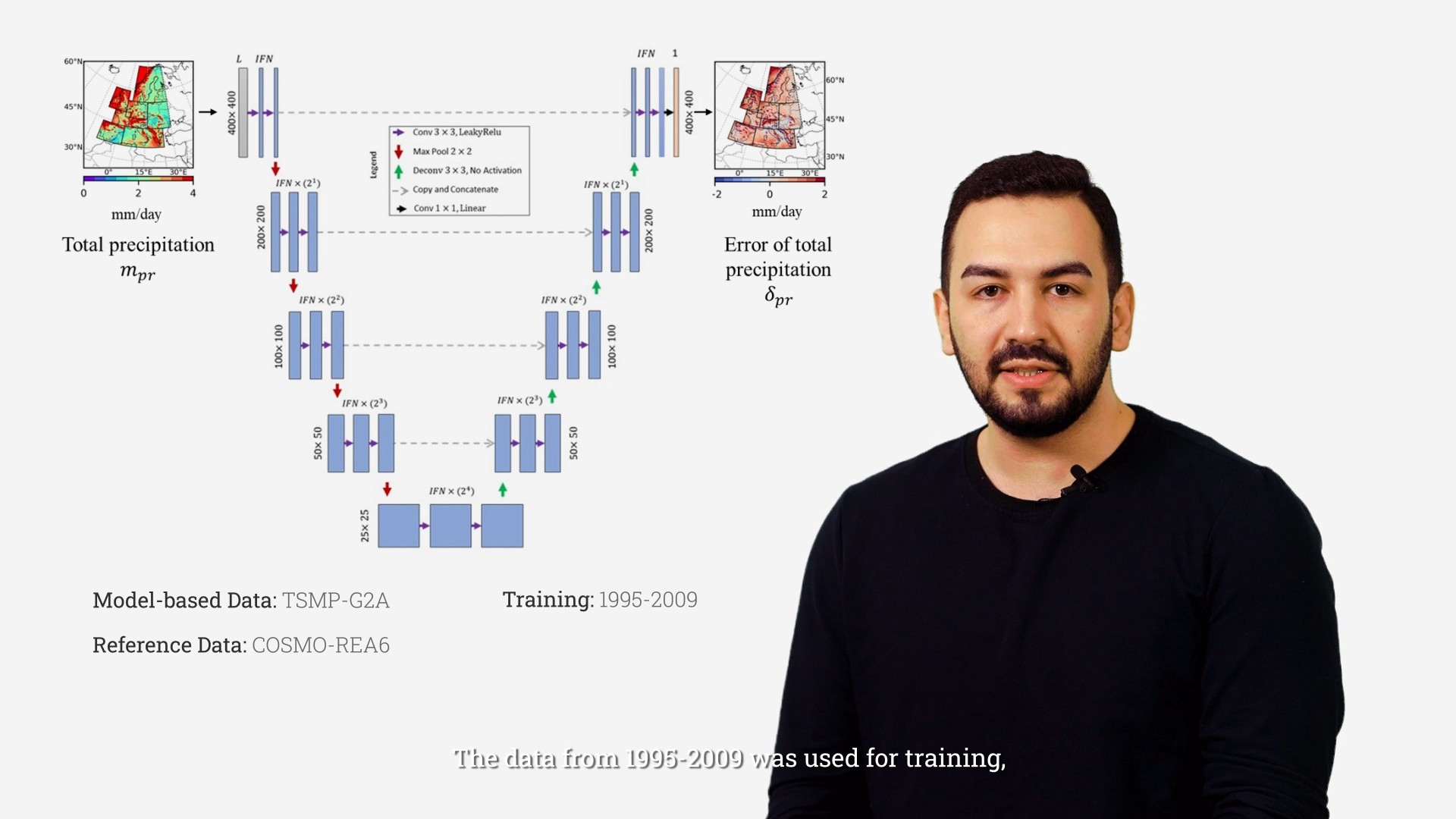
AI for weather modeling
Explore deep learning applications in weather modeling, learn about merging atmospheric model results and observations, and understand how U-Net Convolutional Neural Network (CNN) can be used to improve precipitation predictions.

Explainable machine learning
Master explainable machine learning with basics and advanced methods. Learn how to interpret linear regression, decision trees, and neural networks. Dive into global and local model-agnostic techniques. Explore Shapley values, occlusion sensitivity, and advanced tools.
About
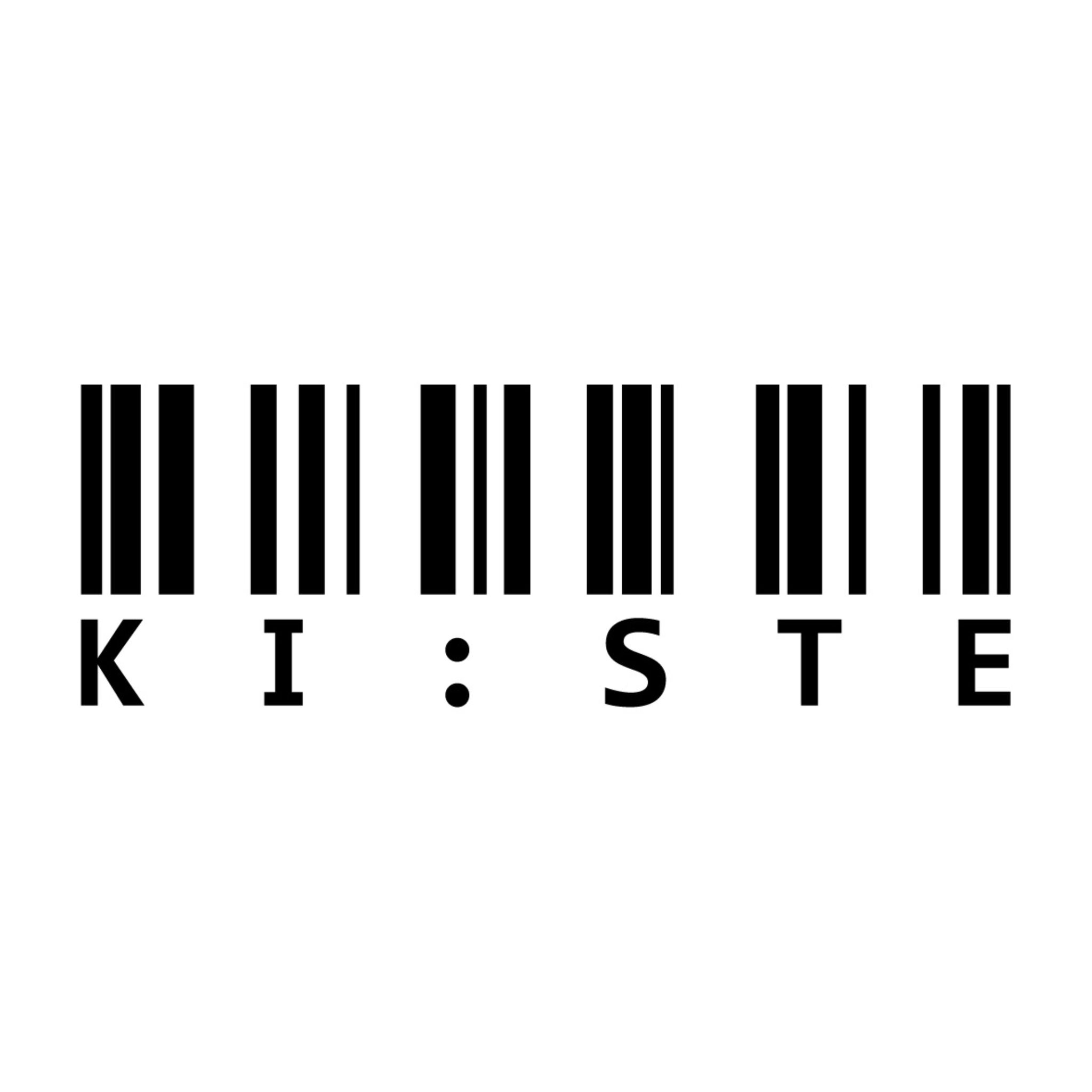
About KI:STE project
Work Packages
Lorem ipsum dolor sit amet, consectetur adipiscing elit. Suspendisse varius enim in eros elementum tristique. Duis cursus, mi quis viverra ornare, eros dolor interdum nulla, ut commodo diam libero vitae erat.
Research
Lorem ipsum dolor sit amet, consectetur adipiscing elit. Suspendisse varius enim in eros elementum tristique. Duis cursus, mi quis viverra ornare, eros dolor interdum nulla, ut commodo diam libero vitae erat.
AI Platform
Lorem ipsum dolor sit amet, consectetur adipiscing elit. Suspendisse varius enim in eros elementum tristique. Duis cursus, mi quis viverra ornare, eros dolor interdum nulla, ut commodo diam libero vitae erat.